Harnessing AI for Advanced Data Analytics and Decision Making
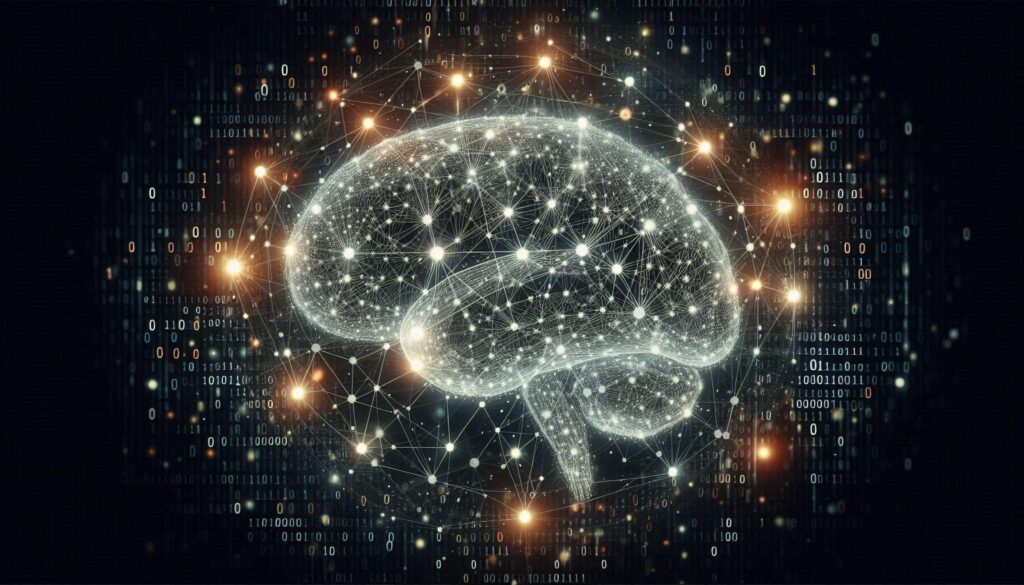
In today’s data-driven world, the integration of artificial intelligence (AI) into data analytics is not just transforming businesses but redefining how decisions are made across industries. Here’s a dive into the more innovative and less commonly discussed aspects of AI in data analytics.
1. Cognitive Analytics for Unstructured Data
Traditional analytics focus heavily on structured data, but the real goldmine lies in unstructured data—text, images, videos, and social media content. AI-powered cognitive analytics can process this unstructured data to extract valuable insights. For example, AI can analyze customer reviews to understand sentiment trends, identify potential product improvements, and foresee market shifts.
2. Automated Data Storytelling
AI doesn’t just analyze data; it can also narrate the findings in human-readable formats. Automated data storytelling tools leverage natural language processing (NLP) to turn complex datasets into coherent, contextually relevant narratives. This is particularly useful for making insights accessible to non-technical stakeholders, enabling better-informed decision-making across all levels of an organization.
3. Edge Analytics
With the proliferation of IoT devices, edge analytics is gaining traction. AI models deployed at the edge—close to the data source—can perform real-time analytics without the latency of cloud processing. This is crucial for industries like manufacturing, where real-time decision-making can prevent equipment failures and optimize operations.
4. AI-Driven Causal Inference
While correlation is useful, understanding causation is vital for strategic decision-making. AI can help distinguish between correlation and causation through advanced techniques like causal inference. For instance, in healthcare, AI can identify which factors genuinely impact patient outcomes, leading to more effective treatments and policies.
5. Synthetic Data for Enhanced Privacy
Data privacy is a significant concern, especially with regulations like GDPR. AI can generate synthetic data that mirrors real-world data without exposing sensitive information. This synthetic data can be used for analysis and model training, ensuring compliance while still leveraging valuable insights.
6. Anomaly Detection in Financial Services
In financial services, anomaly detection is critical for identifying fraudulent activities. AI algorithms can analyze vast datasets to detect unusual patterns that might indicate fraud. For example, AI can monitor transaction data in real-time to flag suspicious activities, enabling quicker response and minimizing financial losses.
7. Predictive Maintenance in Logistics
Logistics companies are using AI to predict equipment failures before they happen. By analyzing data from sensors on vehicles and machinery, AI can forecast when a part is likely to fail and schedule maintenance proactively. This not only reduces downtime but also extends the lifespan of equipment, leading to significant cost savings.
8. AI in Talent Analytics
Human Resources (HR) is leveraging AI to optimize talent management. AI can analyze employee performance data, engagement levels, and even external factors to predict turnover risks and identify high-potential employees. This helps companies to retain top talent and build more effective teams.
Conclusion
AI-driven data analytics is not just about crunching numbers; it’s about transforming raw data into actionable insights that drive strategic decisions. By exploring these advanced applications, businesses can uncover hidden opportunities, mitigate risks, and stay ahead in an increasingly competitive landscape. As AI continues to evolve, its potential to revolutionize data analytics and decision-making will only grow, offering endless possibilities for those willing to innovate and adapt.